Phase 1 - Statistical infrastructure
Authors: Will Chancellor, Shiji Zhao, Lucy Randall, Kenton Lawson and Khanh Hoang
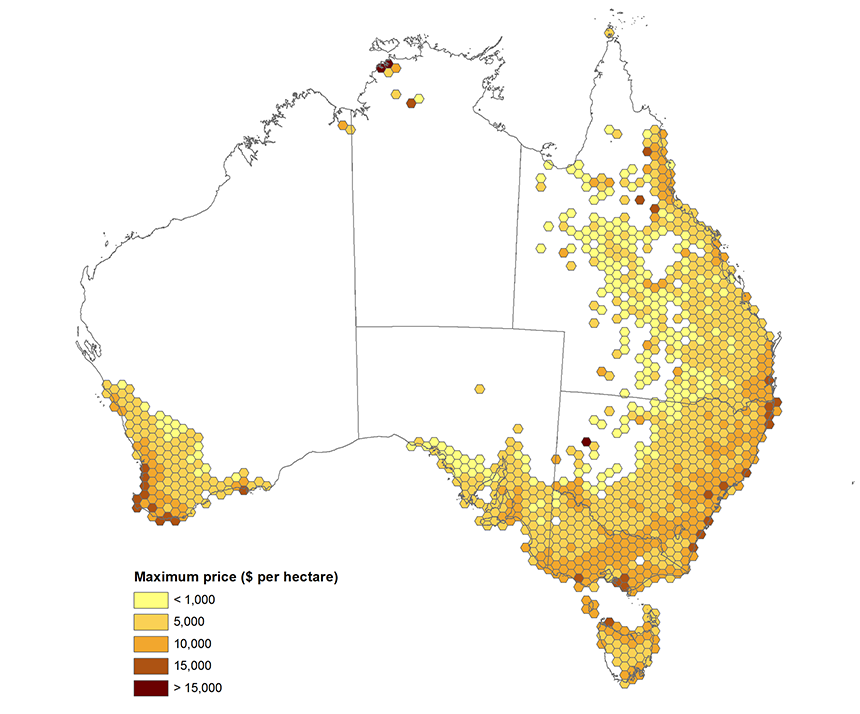
Introduction
Farmland is an important asset for Australian farmers, agricultural industries and the nation as a whole. First, it is a productive asset which is essential for farms to run agricultural businesses, generate revenue and sustain their livelihood. For most farmers, land is their most important asset. Agricultural land is also an integral part of the Australian ecosystem and has a significant environmental value, of which farmers are responsible for managing approximately 385 million hectares (or 58 per cent of Australia).
Information about Australian farmland value is scarce. Values of farms are not observable unless farms are sold on the market and even then, farms are traded infrequently. ABARES produces price indexes of broadacre farmlands (Martin and Topp 2019) for the three ABARES agricultural zones (high rainfall, wheat-sheep and pastoral). However, while these statistics are informative, they have limitations. For example, as the statistics were derived from ABARES Australian Agricultural and Grazing Industry Survey (AAGIS), it is difficult to use the raw data to compile statistics at lower aggregation levels (such as for small farming areas or agricultural regions within a state) due to the limited sample size. Likewise, the index does not cover land used for producing dairy and other agricultural commodities. More importantly, it is not feasible to use the statistics to identify and quantify the determinants of land values (for example, between locations and farm types) and growth over time.
Cost-effective statistical tool
Hedonic models are a feasible and cost-effective statistical tool to fill this information gap. They fit the land value and other data into an equation (or model) using regression or machine learning techniques. In the case of regression, market prices are regressed on the farmland characteristics and the resulting coefficients can be interpreted as the implicit ‘prices’ of the characteristics (embedded in the market prices of farmland). Hence, based on some basic information about the properties (e.g. size and location etc.), a sound hedonic model would enable the estimation of the value of any farmland, including those which have not been sold recently.
While hedonic models have been used extensively to estimate market values of residential properties (Cho 1996; Conniffe and Duffy 1999)1 and consumer goods (Triplett 2004), to our knowledge, comprehensive national hedonic farmland models for Australia do not yet exist. There are several possible explanations for this. The characteristics of farmland are more complex and difficult to identify, define and numerically measure compared to residential properties. There are also many different agricultural production systems, making the stratification of farmland more difficult. Finally, the volume of farmland transactions is smaller compared with the residential market, limiting the applicability of hedonic models to date.
The ‘characteristics’ (physical, natural, geographical and socioeconomic) expected to influence farmland values include terrain features, localised climate conditions (rainfall and temperature), transport access, soil conditions, buildings on the land, production type, among other factors. In addition to these farm specific factors, broader influences such as commodity prices, interest rates, productivity growth, economic growth, market sentiment, levels of rural debt, and the supply and demand for urban real estate assets may also affect the value of farmland.
Using CoreLogic land transaction data and other data ABARES has constructed hedonic models to explain the determinants of the value of agricultural land to estimate values of Australian farmland. The CoreLogic dataset has comprehensive coverage, containing farmland transactions in all States and Territories since 1975 and includes information on transaction values, land size, location, purposes of land use and residential properties on the land (number of bedrooms etc.).
Data transformation
Before constructing the hedonic models, the CoreLogic data required significant data transformation and filtering. The CoreLogic data, contained a total of 700,424 transaction records, including transactions which were obviously not farms. For example, some observations were labelled as ‘construction sites’, ‘mines’ and even ‘church buildings’ etc. Many of the records removed were for agricultural purposes (e.g. dairy, tomato farming, pineapples), however were excluded to fit our scope of dryland broadacre farms. This resulted in a final dataset of 166,994 broadacre farmland transactions covering the period from 1975 to 2019.
The CoreLogic data include information about the location of the land transaction and land parcel identifiers. Using this information, ABARES formed data linkages between these parcel identifiers and state government cadastral datasets, thereby enabling the derivation of parcel shape information for each CoreLogic land transaction record.
The CoreLogic data did not include data on a range of other possible explanatory variables such as climate data, land gradient, water cover and many others. Therefore, ABARES sourced and included data at the farm level on these other possible explanatory variables. The final data set included topography data from the Geoscience Australia Shuttle Radar Topographic Mission (SRTM) digital elevation model, Water Observations from Space (WOS), average rainfall and temperature from the Bureau of Meteorology (BoM) Australian Water Availability Project (AWAP) grids, among others. This means that physical property features (such as soil and climate attributes) can be derived at farm level and applied as explanatory variables to farmland value in the hedonic models.
Applying regressions
After developing the dataset and a prototype model of co-variates to explain our dependent variable (land price per hectare), we apply a series of robust (Hamilton, 1991) and quantile regressions in order to observe the relationship between farmland value and potential explanatory variables by region, production type, size category and price segment. In doing so, we are able to identify some factors driving Australian broadacre farmland value as well as the magnitude and direction of these drivers. This study thereby makes considerable progress in use of hedonic method to measure Australian farmland values and quantify regional, price and size specific drivers.
The hedonic model and underlying data set have a number of potential applications. The underlying data, for example, can be used to generate price index(s) of Australian farmland at the national, state and regional levels, using conventional methods2. The model can be used to identify drivers of farmland price movements at the national, state or regional level as well as predict the impacts of changes in these variables on future farmland prices. For example, it may be possible to estimate the impact of commodity prices and interest rate movements on farmland values.
Future development
Future development of the hedonic models may allow assessments of other policy issues. For example, evaluating the impact of infrastructure investments such as construction of roads, railways and dams on the value of farm land. This could also include assessing the impact of drought and climate change on farmland values. Another possibility is to explore the relationship between natural capital and farm values.
Section 1 presents detailed information about the CoreLogic records, issues with the data and how it is linked to information from other sources. Section 2 explains the hedonic methods used in this preliminary study and Section 3 provides a short summary of the results of hedonic estimation. Section 4 concludes.
Download the full report
Measuring Australian broadacre farmland value: Phase 1 - Statistical infrastructure PDF
Measuring Australian broadacre farmland value: Phase 1 - Statistical infrastructure DOCX